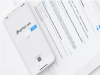
Artificial intelligence in gynecologic cancers: Current status and future challenges - A systematic review.
Akazawa Munetoshi,Hashimoto Kazunori
Artificial intelligence in medicine
OBJECTIVE:Over the past years, the application of artificial intelligence (AI) in medicine has increased rapidly, especially in diagnostics, and in the near future, the role of AI in medicine will become progressively more important. In this study, we elucidated the state of AI research on gynecologic cancers. METHODS:A search was conducted in three databases-PubMed, Web of Science, and Scopus-for research papers dated between January 2010 and December 2020. As keywords, we used "artificial intelligence," "deep learning," "machine learning," and "neural network," combined with "cervical cancer," "endometrial cancer," "uterine cancer," and "ovarian cancer." We excluded genomic and molecular research, as well as automated pap-smear diagnoses and digital colposcopy. RESULTS:Of 1632 articles, 71 were eligible, including 34 on cervical cancer, 13 on endometrial cancer, three on uterine sarcoma, and 21 on ovarian cancer. A total of 35 studies (49%) used imaging data and 36 studies (51%) used value-based data as the input data. Magnetic resonance imaging (MRI), computed tomography (CT), ultrasound, cytology, and hysteroscopy data were used as imaging data, and the patients' backgrounds, blood examinations, tumor markers, and indices in pathological examination were used as value-based data. The targets of prediction were definitive diagnosis and prognostic outcome, including overall survival and lymph node metastasis. The size of the dataset was relatively small because 64 studies (90%) included less than 1000 cases, and the median size was 214 cases. The models were evaluated by accuracy scores, area under the receiver operating curve (AUC), and sensitivity/specificity. Owing to the heterogeneity, a quantitative synthesis was not appropriate in this review. CONCLUSIONS:In gynecologic oncology, more studies have been conducted on cervical cancer than on ovarian and endometrial cancers. Prognoses were mainly used in the study of cervical cancer, whereas diagnoses were primarily used for studying ovarian cancer. The proficiency of the study design for endometrial cancer and uterine sarcoma was unclear because of the small number of studies conducted. The small size of the dataset and the lack of a dataset for external validation were indicated as the challenges of the studies.
10.1016/j.artmed.2021.102164
The challenges of colposcopy for cervical cancer screening in LMICs and solutions by artificial intelligence.
Xue Peng,Ng Man Tat Alexander,Qiao Youlin
BMC medicine
BACKGROUND:The World Health Organization (WHO) called for global action towards the elimination of cervical cancer. One of the main strategies is to screen 70% of women at the age between 35 and 45 years and 90% of women managed appropriately by 2030. So far, approximately 85% of cervical cancers occur in low- and middle-income countries (LMICs). The colposcopy-guided biopsy is crucial for detecting cervical intraepithelial neoplasia (CIN) and becomes the main bottleneck limiting screening performance. Unprecedented advances in artificial intelligence (AI) enable the synergy of deep learning and digital colposcopy, which offers opportunities for automatic image-based diagnosis. To this end, we discuss the main challenges of traditional colposcopy and the solutions applying AI-guided digital colposcopy as an auxiliary diagnostic tool in low- and middle- income countries (LMICs). MAIN BODY:Existing challenges for the application of colposcopy in LMICs include strong dependence on the subjective experience of operators, substantial inter- and intra-operator variabilities, shortage of experienced colposcopists, consummate colposcopy training courses, and uniform diagnostic standard and strict quality control that are hard to be followed by colposcopists with limited diagnostic ability, resulting in discrepant reporting and documentation of colposcopy impressions. Organized colposcopy training courses should be viewed as an effective way to enhance the diagnostic ability of colposcopists, but implementing these courses in practice may not always be feasible to improve the overall diagnostic performance in a short period of time. Fortunately, AI has the potential to address colposcopic bottleneck, which could assist colposcopists in colposcopy imaging judgment, detection of underlying CINs, and guidance of biopsy sites. The automated workflow of colposcopy examination could create a novel cervical cancer screening model, reduce potentially false negatives and false positives, and improve the accuracy of colposcopy diagnosis and cervical biopsy. CONCLUSION:We believe that a practical and accurate AI-guided digital colposcopy has the potential to strengthen the diagnostic ability in guiding cervical biopsy, thereby improves cervical cancer screening performance in LMICs and accelerates the process of global cervical cancer elimination eventually.
10.1186/s12916-020-01613-x