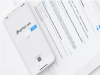
Machine Learning as a Diagnostic and Prognostic Tool for Predicting Thrombosis in Cancer Patients: A Systematic Review.
Seminars in thrombosis and hemostasis
Khorana score (KS) is an established risk assessment model for predicting cancer-associated thrombosis. However, it ignores several risk factors and has poor predictability in some cancer types. Machine learning (ML) is a novel technique used for the diagnosis and prognosis of several diseases, including cancer-associated thrombosis, when trained on specific diagnostic modalities. Consolidating the literature on the use of ML for the prediction of cancer-associated thrombosis is necessary to understand its diagnostic and prognostic abilities relative to KS. This systematic review aims to evaluate the current use and performance of ML algorithms to predict thrombosis in cancer patients. This study was conducted per Preferred Reporting Items for Systematic Reviews and Meta-Analysis guidelines. Databases Medline, EMBASE, Cochrane, and ClinicalTrials.gov, were searched from inception to September 15, 2023, for studies evaluating the use of ML models for the prediction of thrombosis in cancer patients. Search terms "machine learning," "artificial intelligence," "thrombosis," and "cancer" were used. Studies that examined adult cancer patients using any ML model were included. Two independent reviewers conducted study selection and data extraction. Three hundred citations were screened, of which 29 studies underwent a full-text review, and ultimately, 8 studies with 22,893 patients were included. Sample sizes ranged from 348 to 16,407 patients. Thrombosis was characterized as venous thromboembolism ( = 6) or peripherally inserted central catheter thrombosis ( = 2). The types of cancer included breast, gastric, colorectal, bladder, lung, esophageal, pancreatic, biliary, prostate, ovarian, genitourinary, head-neck, and sarcoma. All studies reported outcomes on the ML's predictive capacity. The extreme gradient boosting appears to be the best-performing model, and several models outperform KS in their respective datasets.
10.1055/s-0044-1785482
Machine Learning for Early Discrimination Between Lung Cancer and Benign Nodules Using Routine Clinical and Laboratory Data.
Annals of surgical oncology
BACKGROUND:Lung cancer poses a global health threat necessitating early detection and precise staging for improved patient outcomes. This study focuses on developing and validating a machine learning-based risk model for early lung cancer screening and staging, using routine clinical data. METHODS:Two medical center, observational, retrospective studies were conducted, involving 2312 lung cancer patients and 653 patients with benign nodules. Machine learning techniques, including differential analysis and feature selection, were employed to identify key factors for modeling. The study focused on variables such as nodule density, carcinoembryonic antigen (CEA), age, and lifestyle habits. The Logistic Regression model was utilized for early diagnoses, and the XGBoost model was utilized for staging based on selected features. RESULTS:For early diagnoses, the Logistic Regression model achieved an area under the curve (AUC) of 0.716 (95% confidence interval [CI] 0.607-0.826), with 0.703 sensitivity and 0.654 specificity. The XGBoost model excelled in distinguishing late-stage from early-stage lung cancer, exhibiting an AUC of 0.913 (95% CI 0.862-0.963), with 0.909 sensitivity and 0.814 specificity. These findings highlight the model's potential for enhancing diagnostic accuracy and staging in lung cancer. CONCLUSION:This study introduces a novel machine learning-based risk model for early lung cancer screening and staging, leveraging routine clinical information and laboratory data. The model shows promise in enhancing accuracy, mitigating overdiagnosis, and improving patient outcomes.
10.1245/s10434-024-15762-3
Revolutionizing anemia detection: integrative machine learning models and advanced attention mechanisms.
Visual computing for industry, biomedicine, and art
This study addresses the critical issue of anemia detection using machine learning (ML) techniques. Although a widespread blood disorder with significant health implications, anemia often remains undetected. This necessitates timely and efficient diagnostic methods, as traditional approaches that rely on manual assessment are time-consuming and subjective. The present study explored the application of ML - particularly classification models, such as logistic regression, decision trees, random forest, support vector machines, Naïve Bayes, and k-nearest neighbors - in conjunction with innovative models incorporating attention modules and spatial attention to detect anemia. The proposed models demonstrated promising results, achieving high accuracy, precision, recall, and F1 scores for both textual and image datasets. In addition, an integrated approach that combines textual and image data was found to outperform the individual modalities. Specifically, the proposed AlexNet Multiple Spatial Attention model achieved an exceptional accuracy of 99.58%, emphasizing its potential to revolutionize automated anemia detection. The results of ablation studies confirm the significance of key components - including the blue-green-red, multiple, and spatial attentions - in enhancing model performance. Overall, this study presents a comprehensive and innovative framework for noninvasive anemia detection, contributing valuable insights to the field.
10.1186/s42492-024-00169-4