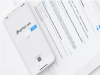
Using digital phenotyping to classify bipolar disorder and unipolar disorder - exploratory findings using machine learning models.
European neuropsychopharmacology : the journal of the European College of Neuropsychopharmacology
The aims were to investigate 1) differences in smartphone-based data on phone usage between bipolar disorder (BD) and unipolar disorder (UD) and 2) by using machine learning models, the sensitivity, specificity, and AUC of the combined smartphone data in classifying BD and UD. Daily smartphone-based self-assessments of mood and same-time passively collected smartphone data on smartphone usage was available for six months. A total of 64 patients with BD and 74 patients with UD were included. Patients with BD during euthymic states compared with UD in euthymic states had a lower number of incoming phone calls/ day (B: -0.70, 95%CI: -1.37; -0.70, p=0.040). Patients with BD during depressive states had a lower number of incoming and outgoing phone calls/ day as compared with patients with UD in depressive states. In classification by using machine learning models, 1) overall (regardless of the affective state), patients with BD were classified with an AUC of 0.84, which reduced to 0.48 when using a leave-one-patient-out crossvalidation (LOOCV) approach; similarly 2) during a depressive state, patients with BD were classified with an AUC of 0.86, which reduced to 0.42 with LOOCV; 3) during a euthymic state, patients with BD were classified with an AUC of 0.87, which reduced to 0.46 with LOOCV. While digital phenotyping shows promise in differentiating between patients with BD and UD, it highlights the challenge of generalizing to unseen individuals. It should serve as an complement to comprehensive clinical evaluation by clinicians.
10.1016/j.euroneuro.2024.01.003
Employing biochemical biomarkers for building decision tree models to predict bipolar disorder from major depressive disorder.
Journal of affective disorders
BACKGROUND:Conventional biochemical parameters may have predictive values for use in clinical identification between bipolar disorder (BD) and major depressive disorder (MDD). METHODS:This study enrolled 2470 hospitalized patients with BD (n = 1333) or MDD (n = 1137) at reproductive age from 2009 to 2018 in China. We extracted 8 parameters, uric acid (UA), direct bilirubin (DBIL), indirect bilirubin (IDBIL), lactic dehydrogenase (LDH), free triiodothyronine (FT3), thyroid-stimulating hormone (TSH), high-density lipoprotein (HDL) and prealbumin of male, patients and 12 parameters, UA, DBIL, IBIL, LDH, FT3, TSH, glutamic-pyruvic transaminase (GPT), white blood cell (WBC), alkaline phosphatase (ALP), fasting blood glucose (FBG), triglyceride and low-density lipoprotein (LDL) of female patients. Backward stepwise multivariate regression analysis and the Chi-Square Automatic Interaction Detection (CHAID) segmentation analysis via SPSS Decision Tree were implemented to define the discrimination of BD and MDD. RESULTS:DBIL was extracted as the first splitting variable, with LDH and IBIL as the second, TSH and prealbumin as the third in the model of male patients (p-value < .05). For the model of female patients, DBIL was also extracted as the first splitting variable, with UA, LDH, and IBIL as the second, triglyceride and FT3 as the third (p-value < .05). The predictive accuracies of the Decision Tree and multiple logistic regression models were similar (74.9% vs 76.9% in males; 74.4% vs 79.5% in females). CONCLUSIONS:This study suggests the value of the Decision Tree models, which employ biochemical parameters as diagnostic predictors for BD and MDD. The CHAID Decision Tree identified that patients with concomitantly increased LDH, IBIL, and decreased DBIL could be in the group that showed the highest risk of being diagnosed as BD.
10.1016/j.jad.2022.03.080