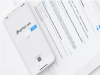
Readability and Information Quality in Cancer Information From a Free vs Paid Chatbot.
JAMA network open
Importance:The mainstream use of chatbots requires a thorough investigation of their readability and quality of information. Objective:To identify readability and quality differences in information between a free and paywalled chatbot cancer-related responses, and to explore if more precise prompting can mitigate any observed differences. Design, Setting, and Participants:This cross-sectional study compared readability and information quality of a chatbot's free vs paywalled responses with Google Trends' top 5 search queries associated with breast, lung, prostate, colorectal, and skin cancers from January 1, 2021, to January 1, 2023. Data were extracted from the search tracker, and responses were produced by free and paywalled ChatGPT. Data were analyzed from December 20, 2023, to January 15, 2024. Exposures:Free vs paywalled chatbot outputs with and without prompt: "Explain the following at a sixth grade reading level: [nonprompted input]." Main Outcomes and Measures:The primary outcome measured the readability of a chatbot's responses using Flesch Reading Ease scores (0 [graduate reading level] to 100 [easy fifth grade reading level]). Secondary outcomes included assessing consumer health information quality with the validated DISCERN instrument (overall score from 1 [low quality] to 5 [high quality]) for each response. Scores were compared between the 2 chatbot models with and without prompting. Results:This study evaluated 100 chatbot responses. Nonprompted free chatbot responses had lower readability (median [IQR] Flesh Reading ease scores, 52.60 [44.54-61.46]) than nonprompted paywalled chatbot responses (62.48 [54.83-68.40]) (P < .05). However, prompting the free chatbot to reword responses at a sixth grade reading level was associated with increased reading ease scores than the paywalled chatbot nonprompted responses (median [IQR], 71.55 [68.20-78.99]) (P < .001). Prompting was associated with increases in reading ease in both free (median [IQR], 71.55 [68.20-78.99]; P < .001)and paywalled versions (median [IQR], 75.64 [70.53-81.12]; P < .001). There was no significant difference in overall DISCERN scores between the chatbot models, with and without prompting. Conclusions and Relevance:In this cross-sectional study, paying for the chatbot was found to provide easier-to-read responses, but prompting the free version of the chatbot was associated with increased response readability without changing information quality. Educating the public on how to prompt chatbots may help promote equitable access to health information.
10.1001/jamanetworkopen.2024.22275
Comparing Physician and Artificial Intelligence Chatbot Responses to Patient Questions Posted to a Public Social Media Forum.
JAMA internal medicine
Importance:The rapid expansion of virtual health care has caused a surge in patient messages concomitant with more work and burnout among health care professionals. Artificial intelligence (AI) assistants could potentially aid in creating answers to patient questions by drafting responses that could be reviewed by clinicians. Objective:To evaluate the ability of an AI chatbot assistant (ChatGPT), released in November 2022, to provide quality and empathetic responses to patient questions. Design, Setting, and Participants:In this cross-sectional study, a public and nonidentifiable database of questions from a public social media forum (Reddit's r/AskDocs) was used to randomly draw 195 exchanges from October 2022 where a verified physician responded to a public question. Chatbot responses were generated by entering the original question into a fresh session (without prior questions having been asked in the session) on December 22 and 23, 2022. The original question along with anonymized and randomly ordered physician and chatbot responses were evaluated in triplicate by a team of licensed health care professionals. Evaluators chose "which response was better" and judged both "the quality of information provided" (very poor, poor, acceptable, good, or very good) and "the empathy or bedside manner provided" (not empathetic, slightly empathetic, moderately empathetic, empathetic, and very empathetic). Mean outcomes were ordered on a 1 to 5 scale and compared between chatbot and physicians. Results:Of the 195 questions and responses, evaluators preferred chatbot responses to physician responses in 78.6% (95% CI, 75.0%-81.8%) of the 585 evaluations. Mean (IQR) physician responses were significantly shorter than chatbot responses (52 [17-62] words vs 211 [168-245] words; t = 25.4; P < .001). Chatbot responses were rated of significantly higher quality than physician responses (t = 13.3; P < .001). The proportion of responses rated as good or very good quality (≥ 4), for instance, was higher for chatbot than physicians (chatbot: 78.5%, 95% CI, 72.3%-84.1%; physicians: 22.1%, 95% CI, 16.4%-28.2%;). This amounted to 3.6 times higher prevalence of good or very good quality responses for the chatbot. Chatbot responses were also rated significantly more empathetic than physician responses (t = 18.9; P < .001). The proportion of responses rated empathetic or very empathetic (≥4) was higher for chatbot than for physicians (physicians: 4.6%, 95% CI, 2.1%-7.7%; chatbot: 45.1%, 95% CI, 38.5%-51.8%; physicians: 4.6%, 95% CI, 2.1%-7.7%). This amounted to 9.8 times higher prevalence of empathetic or very empathetic responses for the chatbot. Conclusions:In this cross-sectional study, a chatbot generated quality and empathetic responses to patient questions posed in an online forum. Further exploration of this technology is warranted in clinical settings, such as using chatbot to draft responses that physicians could then edit. Randomized trials could assess further if using AI assistants might improve responses, lower clinician burnout, and improve patient outcomes.
10.1001/jamainternmed.2023.1838
Physician and Artificial Intelligence Chatbot Responses to Cancer Questions From Social Media.
JAMA oncology
Importance:Artificial intelligence (AI) chatbots pose the opportunity to draft template responses to patient questions. However, the ability of chatbots to generate responses based on domain-specific knowledge of cancer remains to be tested. Objective:To evaluate the competency of AI chatbots (GPT-3.5 [chatbot 1], GPT-4 [chatbot 2], and Claude AI [chatbot 3]) to generate high-quality, empathetic, and readable responses to patient questions about cancer. Design, Setting, and Participants:This equivalence study compared the AI chatbot responses and responses by 6 verified oncologists to 200 patient questions about cancer from a public online forum. Data were collected on May 31, 2023. Exposures:Random sample of 200 patient questions related to cancer from a public online forum (Reddit r/AskDocs) spanning from January 1, 2018, to May 31, 2023, was posed to 3 AI chatbots. Main Outcomes and Measures:The primary outcomes were pilot ratings of the quality, empathy, and readability on a Likert scale from 1 (very poor) to 5 (very good). Two teams of attending oncology specialists evaluated each response based on pilot measures of quality, empathy, and readability in triplicate. The secondary outcome was readability assessed using Flesch-Kincaid Grade Level. Results:Responses to 200 questions generated by chatbot 3, the best-performing AI chatbot, were rated consistently higher in overall measures of quality (mean, 3.56 [95% CI, 3.48-3.63] vs 3.00 [95% CI, 2.91-3.09]; P < .001), empathy (mean, 3.62 [95% CI, 3.53-3.70] vs 2.43 [95% CI, 2.32-2.53]; P < .001), and readability (mean, 3.79 [95% CI, 3.72-3.87] vs 3.07 [95% CI, 3.00-3.15]; P < .001) compared with physician responses. The mean Flesch-Kincaid Grade Level of physician responses (mean, 10.11 [95% CI, 9.21-11.03]) was not significantly different from chatbot 3 responses (mean, 10.31 [95% CI, 9.89-10.72]; P > .99) but was lower than those from chatbot 1 (mean, 12.33 [95% CI, 11.84-12.83]; P < .001) and chatbot 2 (mean, 11.32 [95% CI, 11.05-11.79]; P = .01). Conclusions and Relevance:The findings of this study suggest that chatbots can generate quality, empathetic, and readable responses to patient questions comparable to physician responses sourced from an online forum. Further research is required to assess the scope, process integration, and patient and physician outcomes of chatbot-facilitated interactions.
10.1001/jamaoncol.2024.0836
An artificial intelligence-based chatbot for prostate cancer education: Design and patient evaluation study.
Digital health
Introduction:Artificial intelligence (AI) is increasingly used in healthcare. AI-based chatbots can act as automated conversational agents, capable of promoting health and providing education at any time. The objective of this study was to develop and evaluate a user-friendly medical chatbot (prostate cancer communication assistant (PROSCA)) for provisioning patient information about early detection of prostate cancer (PC). Methods:The chatbot was developed to provide information on prostate diseases, diagnostic tests for PC detection, stages, and treatment options. Ten men aged 49 to 81 years with suspicion of PC were enrolled in this study. Nine of ten patients used the chatbot during the evaluation period and filled out the questionnaires on usage and usability, perceived benefits, and potential for improvement. Results:The chatbot was straightforward to use, with 78% of users not needing any assistance during usage. In total, 89% of the chatbot users in the study experienced a clear to moderate increase in knowledge about PC through the chatbot. All study participants who tested the chatbot would like to re-use a medical chatbot in the future and support the use of chatbots in the clinical routine. Conclusions:Through the introduction of the chatbot PROSCA, we created and evaluated an innovative evidence-based health information tool in the field of PC, allowing targeted support for doctor-patient communication and offering great potential in raising awareness, patient education, and support. Our study revealed that a medical chatbot in the field of early PC detection is readily accepted and benefits patients as an additional informative tool.
10.1177/20552076231173304
Accuracy and Reliability of Chatbot Responses to Physician Questions.
JAMA network open
Importance:Natural language processing tools, such as ChatGPT (generative pretrained transformer, hereafter referred to as chatbot), have the potential to radically enhance the accessibility of medical information for health professionals and patients. Assessing the safety and efficacy of these tools in answering physician-generated questions is critical to determining their suitability in clinical settings, facilitating complex decision-making, and optimizing health care efficiency. Objective:To assess the accuracy and comprehensiveness of chatbot-generated responses to physician-developed medical queries, highlighting the reliability and limitations of artificial intelligence-generated medical information. Design, Setting, and Participants:Thirty-three physicians across 17 specialties generated 284 medical questions that they subjectively classified as easy, medium, or hard with either binary (yes or no) or descriptive answers. The physicians then graded the chatbot-generated answers to these questions for accuracy (6-point Likert scale with 1 being completely incorrect and 6 being completely correct) and completeness (3-point Likert scale, with 1 being incomplete and 3 being complete plus additional context). Scores were summarized with descriptive statistics and compared using the Mann-Whitney U test or the Kruskal-Wallis test. The study (including data analysis) was conducted from January to May 2023. Main Outcomes and Measures:Accuracy, completeness, and consistency over time and between 2 different versions (GPT-3.5 and GPT-4) of chatbot-generated medical responses. Results:Across all questions (n = 284) generated by 33 physicians (31 faculty members and 2 recent graduates from residency or fellowship programs) across 17 specialties, the median accuracy score was 5.5 (IQR, 4.0-6.0) (between almost completely and complete correct) with a mean (SD) score of 4.8 (1.6) (between mostly and almost completely correct). The median completeness score was 3.0 (IQR, 2.0-3.0) (complete and comprehensive) with a mean (SD) score of 2.5 (0.7). For questions rated easy, medium, and hard, the median accuracy scores were 6.0 (IQR, 5.0-6.0), 5.5 (IQR, 5.0-6.0), and 5.0 (IQR, 4.0-6.0), respectively (mean [SD] scores were 5.0 [1.5], 4.7 [1.7], and 4.6 [1.6], respectively; P = .05). Accuracy scores for binary and descriptive questions were similar (median score, 6.0 [IQR, 4.0-6.0] vs 5.0 [IQR, 3.4-6.0]; mean [SD] score, 4.9 [1.6] vs 4.7 [1.6]; P = .07). Of 36 questions with scores of 1.0 to 2.0, 34 were requeried or regraded 8 to 17 days later with substantial improvement (median score 2.0 [IQR, 1.0-3.0] vs 4.0 [IQR, 2.0-5.3]; P < .01). A subset of questions, regardless of initial scores (version 3.5), were regenerated and rescored using version 4 with improvement (mean accuracy [SD] score, 5.2 [1.5] vs 5.7 [0.8]; median score, 6.0 [IQR, 5.0-6.0] for original and 6.0 [IQR, 6.0-6.0] for rescored; P = .002). Conclusions and Relevance:In this cross-sectional study, chatbot generated largely accurate information to diverse medical queries as judged by academic physician specialists with improvement over time, although it had important limitations. Further research and model development are needed to correct inaccuracies and for validation.
10.1001/jamanetworkopen.2023.36483