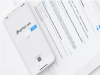
Metabolomics and machine learning approaches for diagnostic and prognostic biomarkers screening in sepsis.
BMC anesthesiology
BACKGROUND:Sepsis is a life-threatening disease with a poor prognosis, and metabolic disorders play a crucial role in its development. This study aims to identify key metabolites that may be associated with the accurate diagnosis and prognosis of sepsis. METHODS:Septic patients and healthy individuals were enrolled to investigate metabolic changes using non-targeted liquid chromatography-high-resolution mass spectrometry metabolomics. Machine learning algorithms were subsequently employed to identify key differentially expressed metabolites (DEMs). Prognostic-related DEMs were then identified using univariate and multivariate Cox regression analyses. The septic rat model was established to verify the effect of phenylalanine metabolism-related gene MAOA on survival and mean arterial pressure after sepsis. RESULTS:A total of 532 DEMs were identified between healthy control and septic patients using metabolomics. The main pathways affected by these DEMs were amino acid biosynthesis, phenylalanine metabolism, tyrosine metabolism, glycine, serine and threonine metabolism, and arginine and proline metabolism. To identify sepsis diagnosis-related biomarkers, support vector machine (SVM) and random forest (RF) algorithms were employed, leading to the identification of four biomarkers. Additionally, analysis of transcriptome data from sepsis patients in the GEO database revealed a significant up-regulation of the phenylalanine metabolism-related gene MAOA in sepsis. Further investigation showed that inhibition of MAOA using the inhibitor RS-8359 reduced phenylalanine levels and improved mean arterial pressure and survival rate in septic rats. Finally, using univariate and multivariate cox regression analysis, six DEMs were identified as prognostic markers for sepsis. CONCLUSIONS:This study employed metabolomics and machine learning algorithms to identify differential metabolites that are associated with the diagnosis and prognosis of sepsis patients. Unraveling the relationship between metabolic characteristics and sepsis provides new insights into the underlying biological mechanisms, which could potentially assist in the diagnosis and treatment of sepsis. TRIAL REGISTRATION:This human study was approved by the Ethics Committee of the Research Institute of Surgery (2021-179) and was registered by the Chinese Clinical Trial Registry (Date: 09/12/2021, ChiCTR2200055772).
10.1186/s12871-023-02317-4
Evaluation of FGFR1 as a diagnostic biomarker for ovarian cancer using TCGA and GEO datasets.
Xiao Huiting,Wang Kun,Li Dan,Wang Ke,Yu Min
PeerJ
Background:Malignant ovarian cancer is associated with the highest mortality of all gynecological tumors. Designing therapeutic targets that are specific to OC tissue is important for optimizing OC therapies. This study aims to identify different expression patterns of genes related to FGFR1 and the usefulness of FGFR1 as diagnostic biomarker for OC. Methods:We collected data from The Cancer Genome Atlas (TCGA) and the Gene Expression Omnibus (GEO) databases. In the TCGA cohort we analyzed clinical information according to patient characteristics, including age, stage, grade, longest dimension of the tumor and the presence of a residual tumor. GEO data served as a validation set. We obtained data on differentially expressed genes (DEGs) from the two microarray datasets. We then used gene set enrichment analysis (GSEA) to analyze the DEG data in order to identify enriched pathways related to FGFR1. Results:Differential expression analysis revealed that FGFR1 was significantly downregulated in OC specimens. 303 patients were included in the TCGA cohort. The GEO dataset confirmed these findings using information on 75 Asian patients. The GSE105437 and GSE12470 database highlighted the significant diagnostic value of FGFR1 in identifying OC (AUC = 1, = 0.0009 and AUC = 0.8256, = 0.0015 respectively). Conclusions:Our study examined existing TCGA and GEO datasets for novel factors associated with OC and identified FGFR1 as a potential diagnostic factor. Further investigation is warranted to characterize the role played by FGFR1 in OC.
10.7717/peerj.10817
NCBI GEO: archive for functional genomics data sets--update.
Barrett Tanya,Wilhite Stephen E,Ledoux Pierre,Evangelista Carlos,Kim Irene F,Tomashevsky Maxim,Marshall Kimberly A,Phillippy Katherine H,Sherman Patti M,Holko Michelle,Yefanov Andrey,Lee Hyeseung,Zhang Naigong,Robertson Cynthia L,Serova Nadezhda,Davis Sean,Soboleva Alexandra
Nucleic acids research
The Gene Expression Omnibus (GEO, http://www.ncbi.nlm.nih.gov/geo/) is an international public repository for high-throughput microarray and next-generation sequence functional genomic data sets submitted by the research community. The resource supports archiving of raw data, processed data and metadata which are indexed, cross-linked and searchable. All data are freely available for download in a variety of formats. GEO also provides several web-based tools and strategies to assist users to query, analyse and visualize data. This article reports current status and recent database developments, including the release of GEO2R, an R-based web application that helps users analyse GEO data.
10.1093/nar/gks1193