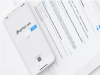
Development and validation of a clinical prediction model to estimate the risk of critical patients with COVID-19.
Journal of medical virology
The outbreak of coronavirus disease 2019 (COVID-19) has globally strained medical resources and caused significant mortality. This study was aimed to develop and validate a prediction model based on clinical features to estimate the risk of patients with COVID-19 at admission progressing to critical patients. Patients admitted to the hospital between January 16, 2020, and March 10, 2020, were retrospectively enrolled, and they were observed for at least 14 days after admission to determine whether they developed into severe pneumonia. According to the clinical symptoms, all patients were divided into four groups: mild, normal, severe, and critical. A total of 390 patients with COVID-19 pneumonia were identified, including 212 severe patients and 178 nonsevere patients. The least absolute shrinkage and selection operator (LASSO) regression reduced the variables in the model to 6, which are age, number of comorbidities, computed tomography severity score, lymphocyte count, aspartate aminotransferase, and albumin. The area under curve of the model in the training set is 0.898, and the specificity and sensitivity were 89.7% and 75.5%. The prediction model, nomogram might be useful to access the onset of severe and critical illness among COVID-19 patients at admission, which is instructive for clinical diagnosis.
10.1002/jmv.27428
Highlighting uncertainty in clinical risk prediction using a model of emergency laparotomy mortality risk.
NPJ digital medicine
Clinical prediction models typically make point estimates of risk. However, values of key variables are often missing during model development or at prediction time, meaning that the point estimates mask significant uncertainty and can lead to over-confident decision making. We present a model of mortality risk in emergency laparotomy which instead presents a distribution of predicted risks, highlighting the uncertainty over the risk of death with an intuitive visualisation. We developed and validated our model using data from 127134 emergency laparotomies from patients in England and Wales during 2013-2019. We captured the uncertainty arising from missing data using multiple imputation, allowing prospective, patient-specific imputation for variables that were frequently missing. Prospective imputation allows early prognostication in patients where these variables are not yet measured, accounting for the additional uncertainty this induces. Our model showed good discrimination and calibration (95% confidence intervals: Brier score 0.071-0.078, C statistic 0.859-0.873, calibration error 0.031-0.059) on unseen data from 37 hospitals, consistently improving upon the current gold-standard model. The dispersion of the predicted risks varied significantly between patients and increased where prospective imputation occurred. We present a case study that illustrates the potential impact of uncertainty quantification on clinical decision making. Our model improves mortality risk prediction in emergency laparotomy and has the potential to inform decision-makers and assist discussions with patients and their families. Our analysis code was robustly developed and is publicly available for easy replication of our study and adaptation to predicting other outcomes.
10.1038/s41746-022-00616-7
Clinical characteristics and mortality risk prediction model in children with acute myocarditis.
World journal of pediatrics : WJP
BACKGROUND:Acute myocarditis (AMC) can cause poor outcomes or even death in children. We aimed to identify AMC risk factors and create a mortality prediction model for AMC in children at hospital admission. METHODS:This was a single-center retrospective cohort study of AMC children hospitalized between January 2016 and January 2020. The demographics, clinical examinations, types of AMC, and laboratory results were collected at hospital admission. In-hospital survival or death was documented. Clinical characteristics associated with death were evaluated. RESULTS:Among 67 children, 51 survived, and 16 died. The most common symptom was digestive disorder (67.2%). Based on the Bayesian model averaging and Hosmer-Lemeshow test, we created a final best mortality prediction model (acute myocarditis death risk score, AMCDRS) that included ten variables (male sex, fever, congestive heart failure, left-ventricular ejection fraction < 50%, pulmonary edema, ventricular tachycardia, lactic acid value > 4, fulminant myocarditis, abnormal creatine kinase-MB, and hypotension). Despite differences in the characteristics of the validation cohort, the model discrimination was only marginally lower, with an AUC of 0.781 (95% confidence interval = 0.675-0.852) compared with the derivation cohort. Model calibration likewise indicated acceptable fit (Hosmer‒Lemeshow goodness-of-fit, P¼ = 0.10). CONCLUSIONS:Multiple factors were associated with increased mortality in children with AMC. The prediction model AMCDRS might be used at hospital admission to accurately identify AMC in children who are at an increased risk of death.
10.1007/s12519-022-00637-y
A clinical prediction model for estimating the risk of developing uveitis in patients with juvenile idiopathic arthritis.
Rheumatology (Oxford, England)
OBJECTIVE:To build a prediction model for uveitis in children with JIA for use in current clinical practice. METHODS:Data from the international observational Pharmachild registry were used. Adjusted risk factors as well as predictors for JIA-associated uveitis (JIA-U) were determined using multivariable logistic regression models. The prediction model was selected based on the Akaike information criterion. Bootstrap resampling was used to adjust the final prediction model for optimism. RESULTS:JIA-U occurred in 1102 of 5529 JIA patients (19.9%). The majority of patients that developed JIA-U were female (74.1%), ANA positive (66.0%) and had oligoarthritis (59.9%). JIA-U was rarely seen in patients with systemic arthritis (0.5%) and RF positive polyarthritis (0.2%). Independent risk factors for JIA-U were ANA positivity [odds ratio (OR): 1.88 (95% CI: 1.54, 2.30)] and HLA-B27 positivity [OR: 1.48 (95% CI: 1.12, 1.95)] while older age at JIA onset was an independent protective factor [OR: 0.84 (9%% CI: 0.81, 0.87)]. On multivariable analysis, the combination of age at JIA onset [OR: 0.84 (95% CI: 0.82, 0.86)], JIA category and ANA positivity [OR: 2.02 (95% CI: 1.73, 2.36)] had the highest discriminative power among the prediction models considered (optimism-adjusted area under the receiver operating characteristic curve = 0.75). CONCLUSION:We developed an easy to read model for individual patients with JIA to inform patients/parents on the probability of developing uveitis.
10.1093/rheumatology/keaa733
External validation of a biomarker and clinical prediction model for hospital mortality in acute respiratory distress syndrome.
Intensive care medicine
PURPOSE:Mortality prediction in ARDS is important for prognostication and risk stratification. However, no prediction models have been independently validated. A combination of two biomarkers with age and APACHE III was superior in predicting mortality in the NHLBI ARDSNet ALVEOLI trial. We validated this prediction tool in two clinical trials and an observational cohort. METHODS:The validation cohorts included 849 patients from the NHLBI ARDSNet Fluid and Catheter Treatment Trial (FACTT), 144 patients from a clinical trial of sivelestat for ARDS (STRIVE), and 545 ARDS patients from the VALID observational cohort study. To evaluate the performance of the prediction model, the area under the receiver operating characteristic curve (AUC), model discrimination, and calibration were assessed, and recalibration methods were applied. RESULTS:The biomarker/clinical prediction model performed well in all cohorts. Performance was better in the clinical trials with an AUC of 0.74 (95% CI 0.70-0.79) in FACTT, compared to 0.72 (95% CI 0.67-0.77) in VALID, a more heterogeneous observational cohort. The AUC was 0.73 (95% CI 0.70-0.76) when FACTT and VALID were combined. CONCLUSION:We validated a mortality prediction model for ARDS that includes age, APACHE III, surfactant protein D, and interleukin-8 in a variety of clinical settings. Although the model performance as measured by AUC was lower than in the original model derivation cohort, the biomarker/clinical model still performed well and may be useful for risk assessment for clinical trial enrollment, an issue of increasing importance as ARDS mortality declines, and better methods are needed for selection of the most severely ill patients for inclusion.
10.1007/s00134-017-4854-5
Prediction model for knee osteoarthritis based on genetic and clinical information.
Arthritis research & therapy
INTRODUCTION:Osteoarthritis (OA) is the most common bone and joint disease influenced by genetic and environmental factors. Recent association studies have uncovered the genetic factors behind OA, its susceptibility genes, which would enable us to predict disease occurrence based on genotype information. However, most previous studies have evaluated the effects of only a single susceptibility gene, and hence prediction based on such information is not as reliable. Here, we constructed OA-prediction models based on genotype information from a case-control association study and tested their predictability. METHODS:We genotyped risk alleles of the three susceptibility genes, asporin (ASPN), growth differentiation factor 5 (GDF5), and double von Willebrand factor A domains (DVWA) for a total of 2,158 Japanese subjects (933 OA and 1,225 controls) and statistically analyzed their effects. After that, we constructed prediction models by using the logistic regression analysis. RESULTS:When the effects of each allele were assumed to be the same and multiplicative, each additional risk allele increased the odds ratio (OR) by a factor of 1.23 (95% confidence interval (CI), 1.12 to 1.34). Individuals with five or six risk alleles showed significantly higher susceptibility when compared with those with zero or one, with an OR of 2.67 (95% CI, 1.46 to 4.87; P = 0.0020). Statistical evaluation of the prediction power of models showed that a model using only genotyping data had poor predictability. We obtained a model with good predictability by incorporating clinical data, which was further improved by rigorous age adjustment. CONCLUSIONS:Our results showed that consideration of adjusted clinical information, as well as increases in the number of risk alleles to be integrated, is critical for OA prediction by using data from case-control studies. To the authors' knowledge, this is the first report of the OA-prediction model combining both genetic and clinical information.
10.1186/ar3157
Genetic and clinical risk prediction model for postoperative atrial fibrillation.
Circulation. Arrhythmia and electrophysiology
BACKGROUND:Postoperative atrial fibrillation (PoAF) is common after coronary artery bypass grafting. We previously showed that atrial fibrillation susceptibility single nucleotide polymorphisms (SNPs) at the chromosome 4q25 locus are associated with PoAF. Here, we tested the hypothesis that a combined clinical and genetic model incorporating atrial fibrillation risk SNPs would be superior to a clinical-only model. METHODS AND RESULTS:We developed and externally validated clinical and clinical/genetic risk models for PoAF. The discovery and validation cohorts included 556 and 1164 patients, respectively. Clinical variables previously associated with PoAF and 13 SNPs at loci associated with atrial fibrillation in genome-wide association studies were considered. PoAF occurred in 30% and 29% of patients in the discovery and validation cohorts, respectively. In the discovery cohort, a logistic regression model with clinical factors had good discrimination, with an area under the receiver operator characteristic curve of 0.76. The addition of 10 SNPs to the clinical model did not improve discrimination (area under receiver operator characteristic curve, 0.78; P=0.14 for difference between the 2 models). In the validation cohort, the clinical model had good discrimination (area under the receiver operator characteristic curve, 0.69) and addition of genetic variables resulted in a marginal improvement in discrimination (area under receiver operator characteristic curve, 0.72; P<0.0001). CONCLUSIONS:We developed and validated a model for the prediction of PoAF containing common clinical variables. Addition of atrial fibrillation susceptibility SNPs did not improve model performance. Tools to accurately predict PoAF are needed to risk stratify patients undergoing coronary artery bypass grafting and identify candidates for prophylactic therapies.
10.1161/CIRCEP.114.002300
Solitary pulmonary nodules: clinical prediction model versus physicians.
Swensen S J,Silverstein M D,Edell E S,Trastek V F,Aughenbaugh G L,Ilstrup D M,Schleck C D
Mayo Clinic proceedings
OBJECTIVE:To determine whether a clinical prediction model developed to identify malignant lung nodules based on clinical data and radiologic lung nodule characteristics could predict a malignant lung nodule diagnosis with higher accuracy than physicians. MATERIAL AND METHODS:One hundred cases were obtained by using a stratified random sample from a retrospective cohort of 629 patients with newly discovered 4- to 30-mm radiologically indeterminate solitary pulmonary nodules (SPNs) on chest radiography. A chest radiologist, pulmonologist, thoracic surgeon, and general internist made predictions of a malignant lesion and recommendations for management (thoracotomy, transthoracic needle aspiration biopsy, or observation) on the basis of radiologic and clinical data used to develop the clinical prediction rule. The predictions of a malignant lung nodule were compared with the probability of malignant involvement from a previously validated clinical prediction model to identify malignant nodules on the basis of three clinical characteristics (age, smoking status, and history of cancer greater than or equal to 5 years previously) and three radiologic characteristics (nodule diameter, spiculation, and upper lobe location). RESULTS:Receiver operating characteristic analysis showed no significant difference between the logistic model and the physicians' predictions. Calibration curves revealed that physicians overestimated the probability of a malignant lesion in patients with low risk of malignant disease by the prediction rule; this finding suggests a potential for the decision rule to improve the management of patients with SPNs that are likely to be benign. CONCLUSION:The prediction model was not better than physicians' predictions of malignant SPNs. The prediction rule may have potential to improve the management of patients with SPNs that are likely to be benign.
10.4065/74.4.319
Prediction and clinical utility of a contralateral breast cancer risk model.
Breast cancer research : BCR
BACKGROUND:Breast cancer survivors are at risk for contralateral breast cancer (CBC), with the consequent burden of further treatment and potentially less favorable prognosis. We aimed to develop and validate a CBC risk prediction model and evaluate its applicability for clinical decision-making. METHODS:We included data of 132,756 invasive non-metastatic breast cancer patients from 20 studies with 4682 CBC events and a median follow-up of 8.8 years. We developed a multivariable Fine and Gray prediction model (PredictCBC-1A) including patient, primary tumor, and treatment characteristics and BRCA1/2 germline mutation status, accounting for the competing risks of death and distant metastasis. We also developed a model without BRCA1/2 mutation status (PredictCBC-1B) since this information was available for only 6% of patients and is routinely unavailable in the general breast cancer population. Prediction performance was evaluated using calibration and discrimination, calculated by a time-dependent area under the curve (AUC) at 5 and 10 years after diagnosis of primary breast cancer, and an internal-external cross-validation procedure. Decision curve analysis was performed to evaluate the net benefit of the model to quantify clinical utility. RESULTS:In the multivariable model, BRCA1/2 germline mutation status, family history, and systemic adjuvant treatment showed the strongest associations with CBC risk. The AUC of PredictCBC-1A was 0.63 (95% prediction interval (PI) at 5 years, 0.52-0.74; at 10 years, 0.53-0.72). Calibration-in-the-large was -0.13 (95% PI: -1.62-1.37), and the calibration slope was 0.90 (95% PI: 0.73-1.08). The AUC of Predict-1B at 10 years was 0.59 (95% PI: 0.52-0.66); calibration was slightly lower. Decision curve analysis for preventive contralateral mastectomy showed potential clinical utility of PredictCBC-1A between thresholds of 4-10% 10-year CBC risk for BRCA1/2 mutation carriers and non-carriers. CONCLUSIONS:We developed a reasonably calibrated model to predict the risk of CBC in women of European-descent; however, prediction accuracy was moderate. Our model shows potential for improved risk counseling, but decision-making regarding contralateral preventive mastectomy, especially in the general breast cancer population where limited information of the mutation status in BRCA1/2 is available, remains challenging.
10.1186/s13058-019-1221-1
Development of a clinical prediction model for diagnosing adenomyosis.
Tellum Tina,Nygaard Staale,Skovholt Else K,Qvigstad Erik,Lieng Marit
Fertility and sterility
OBJECTIVE:To develop a multivariate prediction model for diagnosing adenomyosis using predictors available through transvaginal ultrasonography and clinical examinations. DESIGN:Prospective observational single-center study. SETTING:Teaching university hospital. PATIENT(S):One hundred consecutively enrolled premenopausal women aged 30-50 years, undergoing hysterectomy due to a benign condition and not using hormonal treatment. INTERVENTION(S):Preoperative 2-D and 3-D transvaginal ultrasonography investigations were performed, and the results were documented in a standardized form. Clinical information was collected using a questionnaire. Histopathology confirmed the outcome. MAIN OUTCOME MEASURE(S):Diagnostic performance (sensitivity, specificity, area under the curve (AUC)) of a multivariate prediction model for adenomyosis. Independent diagnostic performance of single predictors and their quantitative effect (β) in the final model. RESULT(S):The final model showed a good test quality (area under the curve [AUC] = 0.86, [95% confidence interval = 0.79-0.94], optimal cutoff 0.56, sensitivity of 85%, specificity 78%). The following nine predictors were included ([sensitivity, specificity, β] or [AUC, β]): presence of myometrial cysts (51%, 86%, β = 0.86), fan-shaped echo (36%, 92%, β = 0.54), hyperechoic islets (51%, 78%, β = 0.62), globular uterus (61%, 83%, β = 0.2), normal uterine shape (83%, 61%, β = -0.75), thickest/thinnest ratio for uterine wall (0.61, β = 0.26), maximum width of the junctional zone in sagittal plane (0.71, β = 0.1), regular appearance of junctional zone (31%, 92%, β = -1.0), and grade of dysmenorrhea measured on a verbal numerical rating scale (0.61, β = 0.08). CONCLUSION(S):We have presented a multivariate model for diagnosing adenomyosis that weights predictors based on their diagnostic significance. The reported findings could aid clinicians who are interpreting the heterogeneous appearance of adenomyosis in ultrasonography. CLINICAL TRIAL REGISTRATION NUMBER:NCT02201719.
10.1016/j.fertnstert.2018.06.009
Development, validation and clinical utility of a risk prediction model for adverse pregnancy outcomes in women with gestational diabetes: The PeRSonal GDM model.
EClinicalMedicine
Background:The ability to calculate the absolute risk of adverse pregnancy outcomes for an individual woman with gestational diabetes mellitus (GDM) would allow preventative and therapeutic interventions to be delivered to women at high-risk, sparing women at low-risk from unnecessary care. We aimed to develop, validate and evaluate the clinical utility of a prediction model for adverse pregnancy outcomes in women with GDM. Methods:A prediction model development and validation study was conducted on data from a observational cohort. Participants included all women with GDM from three metropolitan tertiary teaching hospitals in Melbourne, Australia. The development cohort comprised those who delivered between 1 July 2017 to 30 June 2018 and the validation cohort those who delivered between 1 July 2018 to 31 December 2018. The main outcome was a composite of critically important maternal and perinatal complications (hypertensive disorders of pregnancy, large-for-gestational age neonate, neonatal hypoglycaemia requiring intravenous therapy, shoulder dystocia, perinatal death, neonatal bone fracture and nerve palsy). Model performance was measured in terms of discrimination and calibration and clinical utility evaluated using decision curve analysis. Findings:The final PeRSonal (Prediction for Risk Stratified care for women with GDM) model included body mass index, maternal age, fasting and 1-hour glucose values (75-g oral glucose tolerance test), gestational age at GDM diagnosis, Southern and Central Asian ethnicity, East Asian ethnicity, nulliparity, past delivery of an large-for-gestational age neonate, past pre-eclampsia, GWG until GDM diagnosis, and family history of diabetes. The composite adverse pregnancy outcome occurred in 27% (476/1747) of women in the development (1747 women) and in 26% (244/955) in the validation (955 women) cohorts. The model showed excellent calibration with slope of 0.99 (95% CI 0.75 to 1.23) and acceptable discrimination (statistic 0.68; 95% CI 0.64 to 0.72) when temporally validated. Decision curve analysis demonstrated that the model was useful across a range of predicted probability thresholds between 0.15 and 0.85 for adverse pregnancy outcomes compared to the alternatives of managing all women with GDM as if they will or will not have an adverse pregnancy outcome. Interpretation:The PeRSonal GDM model comprising of routinely available clinical data shows compelling performance, is transportable across time, and has clinical utility across a range of predicted probabilities. Further external validation of the model to a more disparate population is now needed to assess the generalisability to different centres, community based care and low resource settings, other healthcare systems and to different GDM diagnostic criteria. Funding:This work is supported by the Mothers and Gestational Diabetes in Australia 2 NHMRC funded project #1170847.
10.1016/j.eclinm.2022.101637
Interpretable clinical visualization model for prediction of prognosis in osteosarcoma: a large cohort data study.
Frontiers in oncology
Background:Currently, the clinical prediction model for patients with osteosarcoma was almost developed from single-center data, lacking external validation. Due to their low reliability and low predictive power, there were few clinical applications. Our study aimed to set up a clinical prediction model with stronger predictive ability, credibility, and clinical application value for osteosarcoma. Methods:Clinical information related to osteosarcoma patients from 2010 to 2016 was collected in the SEER database and four different Chinese medical centers. Factors were screened using three models (full subset regression, univariate Cox, and LASSO) minimum AIC and maximum AUC values in the SEER database. The model was selected by the strongest predictive power and visualized by three statistical methods: nomogram, web calculator, and decision tree. The model was further externally validated and evaluated for its clinical utility in data from four medical centers. Results:Eight predicting factors, namely, age, grade, laterality, stage M, surgery, bone metastases, lung metastases, and tumor size, were selected from the model based on the minimum AIC and maximum AUC value. The internal and external validation results showed that the model possessed good consistency. ROC curves revealed good predictive ability (AUC > 0.8 in both internal and external validation). The DCA results demonstrated that the model had an excellent clinical predicted utility in 3 years and 5 years for North American and Chinese patients. Conclusions:The clinical prediction model was built and visualized in this study, including a nomogram and a web calculator (https://dr-lee.shinyapps.io/osteosarcoma/), which indicated very good consistency, predictive power, and clinical application value.
10.3389/fonc.2022.945362
An early prediction model for gestational diabetes mellitus based on genetic variants and clinical characteristics in China.
Wu Qi,Chen Yanmin,Zhou Menglin,Liu Mengting,Zhang Lixia,Liang Zhaoxia,Chen Danqing
Diabetology & metabolic syndrome
OBJECTIVES:To evaluate the influence of genetic variants and clinical characteristics on the risk of gestational diabetes mellitus (GDM) and to construct and verify a prediction model of GDM in early pregnancy. METHODS:Four hundred seventy five women with GDM and 487 women without, as a control, were included to construct the prediction model of GDM in early pregnancy. Both groups had complete genotyping results and clinical data. They were randomly divided into a trial cohort (70%) and a test cohort (30%). Then, the model validation cohort, including 985 pregnant women, was used for the external validation of the GDM early pregnancy prediction model. RESULTS:We found maternal age, gravidity, parity, BMI and family history of diabetes were significantly associated with GDM (OR > 1; P < 0.001), and assisted reproduction was a critical risk factor for GDM (OR = 1.553, P = 0.055). MTNR1B rs10830963, C2CD4A/B rs1436953 and rs7172432, CMIP rs16955379 were significantly correlated with the incidence of GDM (AOR > 1, P < 0.05). Therefore, these four genetic susceptible single nucleotide polymorphisms (SNPs) and six clinical characteristics were included in the construction of the GDM early pregnancy prediction model. In the trial cohort, a predictive model of GDM in early pregnancy was constructed, in which genetic risk score was independently associated with GDM (AOR = 2.061, P < 0.001) and was the most effective predictor with the exception of family history of diabetes. The ROC-AUC of the prediction model was 0.727 (95% CI 0.690-0.765), and the sensitivity and specificity were 69.9% and 64.0%, respectively. The predictive power was also verified in the test cohort and the validation cohort. CONCLUSIONS:Based on the genetic variants and clinical characteristics, this study developed and verified the early pregnancy prediction model of GDM. This model can help screen out the population at high-risk for GDM in early pregnancy, and lifestyle interventions can be performed for them in a timely manner in early pregnancy.
10.1186/s13098-022-00788-y
A clinical prediction model for cancer-associated venous thromboembolism: a development and validation study in two independent prospective cohorts.
Pabinger Ingrid,van Es Nick,Heinze Georg,Posch Florian,Riedl Julia,Reitter Eva-Maria,Di Nisio Marcello,Cesarman-Maus Gabriela,Kraaijpoel Noémie,Zielinski Christoph Carl,Büller Harry Roger,Ay Cihan
The Lancet. Haematology
BACKGROUND:Venous thromboembolism is a common complication of cancer, but the risk of developing venous thromboembolism varies greatly among individuals and depends on numerous factors, including type of cancer. We aimed to develop and externally validate a clinical prediction model for cancer-associated venous thromboembolism. METHODS:We used data from the prospective Vienna Cancer and Thrombosis Study (CATS) cohort (n=1423) to select prognostic variables for inclusion in the model. We then validated the model in the prospective Multinational Cohort Study to Identify Cancer Patients at High Risk of Venous Thromboembolism (MICA) cohort (n=832). We calculated c-indices to show how the predicted incidence of objectively confirmed venous thromboembolism at 6 months compared with the cumulative 6-month incidences observed in both cohorts. FINDINGS:Two variables were selected for inclusion in the final clinical prediction model: tumour-site risk category (low or intermediate vs high vs very high) and continuous D-dimer concentrations. The multivariable subdistribution hazard ratios were 1·96 (95% CI 1·41-2·72; p=0·0001) for high or very high versus low or intermediate and 1·32 (95% CI 1·12-1·56; p=0·001) per doubling of D-dimer concentration. The cross-validated c-indices of the final model were 0·66 (95% CI 0·63-0·67) in CATS and 0·68 (0·62-0·74) in MICA. The clinical prediction model was adequately calibrated in both cohorts. INTERPRETATION:An externally validated clinical prediction model incorporating only one clinical factor (tumour-site category) and one biomarker (D-dimer) predicted the risk of venous thromboembolism in ambulatory patients with solid cancers. This simple model is a considerable improvement on previous models for predicting cancer-associated venous thromboembolism, and could aid physicians in selection of patients who will likely benefit from thromboprophylaxis. FUNDING:Austrian Science Fund, Austrian National Bank Memorial Fund, and participating hospitals.
10.1016/S2352-3026(18)30063-2
A nomogram prediction model for recurrent laryngeal nerve lymph node metastasis in thoracic oesophageal squamous cell carcinoma.
Journal of thoracic disease
BACKGROUND:The metastasis rate to the recurrent laryngeal nerve lymph node (RLN LN) is high, but resection of it is challenging and increases complications. This study explored the risk factors for the RLN LN metastasis in thoracic oesophageal squamous cell carcinoma and developed a novel scoring system to predict it. METHODS:We retrospectively analysed the clinicopathological data of 265 patients between 2015 and 2018. Univariate and multivariate analyses were performed to screen for risk factors and establish a logistic regression model to predict the risk of RLN LN metastasis. A nomogram was constructed accordingly. Further analyses were conducted regarding right and left RLN LN metastasis alone. RESULTS:(I) The metastatic rates of the left and right RLN LN were 15.1% and 20.4%, respectively. (II) Multivariate logistic regression analysis showed that the short axis diameter of the left RLN LN, short axis diameter of the right RLN LN, maximum diameter of the tumor, tumor location, subcarinal lymph node status and paraoesophageal lymph node status were all independent risk factors for RLN LN metastasis. (III) Multivariate logistic regression analysis showed that the short axis diameter of right RLN LN, tumor location and subcarinal lymph node status were independent risk factors for right RLN LN metastasis. (IV) Multivariate logistic regression analysis showed that short axis diameter of left RLN LN was an independent risk factor for left RLN LN metastasis. CONCLUSIONS:The metastatic rates of the left and right RLN LNs were high and can be predicted according to these nomograms.
10.21037/jtd.2019.06.46
Establishing a prediction model for prostate cancer bone metastasis.
Chen Song,Wang Lu,Qian Kaiyu,Jiang Wei,Deng Haiqing,Zhou Qiang,Wang Gang,Liu Xuefeng,Wu Chin-Lee,Xiao Yu,Wang Xinghuan
International journal of biological sciences
We collected clinical data from 308 prostate cancer (PCa) patients to investigate the clinical characteristics and independent risk factors of bone metastasis (BM) and to establish a prediction model for BM of PCa and determine the necessity of bone scans. Univariate and multivariate analyses were performed based on age, biopsy Gleason score (BGS), clinical tumor stage (cTx), total prostate specific antigen (tPSA), free prostate specific antigen (fPSA), fPSA/tPSA, prostate volume, alkaline phosphatase (ALP), serum calcium and serum phosphorus. Moreover, 80 of the 308 PCa patients had a PI-RADS v2 score and were analysed retrospectively. The univariate analysis showed that the BGS, cTx, tPSA, fPSA, prostate volume and ALP were significant. The multivariate logistic regression analysis showed significant differences among the BGS, cTx, tPSA and ALP. Four cases should be highly suspected with BM: (i) cTl-cT2, BGS ≤7, ALP >120 U/L and tPSA >90.64 ng/ml; (ii) cTl-cT2, BGS ≥8, and ALP >120 U/L; (iii) cT3-cT4, BGS ≤7, and ALP >120 U/L; and (iv) cT3-cT4 and BGS ≥8. After the PI-RADS v2 score was included in the model, the AUC of the prediction model rose from 0.884 (95% CI: 0.813-0.996) to 0.934 (95% CI: 0.883-0.986). This model may help determine the necessity of bone scans to diagnose BM for PCa patients.
10.7150/ijbs.27537
Risk prediction for patients with hepatocellular carcinoma undergoing chemoembolization: development of a prediction model.
Kim Beom Kyung,Shim Ju Hyun,Kim Seung Up,Park Jun Yong,Kim Do Young,Ahn Sang Hoon,Kim Kang Mo,Lim Young-Suk,Han Kwang-Hyub,Lee Han Chu
Liver international : official journal of the International Association for the Study of the Liver
BACKGROUNDS & AIMS:We aimed to generate and validate a novel risk prediction model for patients with hepatocellular carcinoma (HCC) undergoing transarterial chemoembolization (TACE). METHODS:Patients receiving TACE as the first-line therapy between 2006 and 2009 were selected from the databases of two major tertiary hospitals in Korea. This study population was randomly assigned into training (n = 340) and validation (n = 145) sets. From a multivariate Cox-regression model for overall survival (OS), tumour Size, tumour Number, baseline Alpha-foetoprotein level, Child-Pugh class and Objective radiological Response after the first TACE session were selected and then scored to generate a 10-point risk prediction model (named as "SNACOR" model) in the training set. Thereafter, the prognostic performance was assessed in the validation set. RESULTS:In the training set, the time-dependent areas under receiver-operating characteristic curves (AUROCs) for OS at 1-, 3- and 6-years were 0.756, 0.754 and 0.742 respectively. According to the score of the SNACOR model, patients were stratified into three groups; low- (score 0-2), intermediate- (score 3-6) and high-risk group (score 7-10) respectively. The low-risk group had the longest median OS (49.8 months), followed by intermediate- (30.7 months) and high-risk group (12.4 months) (log-rank test, P < 0.001). Compared with the low-risk group, the intermediate-risk (hazard ratio [HR] 2.13, P < 0.001) and high-risk group (HR 6.17, P < 0.001) retained significant risks of death. Similar results were obtained in the validation set. CONCLUSION:A simple-to-use SNACOR model for patients with HCC treated with TACE might be helpful in appropriate prognostification and guidance for decision of further treatment strategies.
10.1111/liv.12865
Risk-prediction Model for Patients Undergoing Laparoscopic Hysterectomy.
Pepin Kristen,Cook Francis,Maghsoudlou Parmida,Cohen Sarah L
Journal of minimally invasive gynecology
STUDY OBJECTIVE:Develop a model for predicting adverse outcomes at the time of laparoscopic hysterectomy (LH) for benign indications. DESIGN:Retrospective cohort study. SETTING:Large academic center. PATIENTS:All patients undergoing LH for benign indications at our institution between 2009 and 2017. INTERVENTIONS:LH (including robot-assisted and laparoscopically assisted vaginal hysterectomy) was performed per standard technique. Data about the patient, surgeon, perioperative adverse outcomes (intraoperative complications, readmission, reoperation, operative time >4 hours, and postoperative medical complications or length of stay >2 days), and uterine weight were collected retrospectively. Pathologic uterine weight was used as a surrogate for predicted preoperative uterine weight. The sample was randomly split, using a random sequence generator, into 2 cohorts, one for deriving the model and the other to validate the model. MEASUREMENTS AND MAIN RESULTS:A total of 3441 patients were included. The rate of composite adverse outcomes was 14.1%. The final logistic regression risk-prediction model identified 6 variables predictive of an adverse outcome at the time of LH: race, history of laparotomy, history of laparoscopy, predicted preoperative uterine weight, body mass index, and surgeon annual case volume. Specifically included were race (97% increased odds of an adverse outcome for black women [95% confidence interval (CI), 34%-110%] and 34% increased odds of an adverse outcome for women of other races [95% CI, -11% to 104%] when compared with white women), history of laparotomy (69% increased odds of an adverse outcome [95% CI, 26%-128%]), history of laparoscopy (65% increased odds of an adverse outcome [95% CI, 21%-124%]), and predicted preoperative uterine weight (2.9% increased odds of an adverse outcome for each 100-g increase in predicted weight [95% CI, 2%-4%]). Body mass index and surgeon annual case volume also had a statistically significant nonlinear relationship with the risk of an adverse outcome. The c-statistic values for the derivation and validation cohorts were 0.74 and 0.72, respectively. The model is best calibrated for patients at lower risk (<20%). CONCLUSION:The LH risk-prediction model is a potentially powerful tool for predicting adverse outcomes in patients planning hysterectomy.
10.1016/j.jmig.2021.03.001
Construction of survival prediction model for elderly esophageal cancer.
Frontiers in oncology
Background:The purpose of this study was to analyze the clinical characteristics and prognosis of EPEC and to construct a prediction model based on the SEER database. Methods:All EPECs from the SEER database were retrospectively analyzed. A comprehensive and practical nomogram that predicts the overall survival (OS) of EPEC was constructed. Univariate and multivariate Cox regression analysis was performed to explore the clinical factors influencing the prognosis of EPEC, and finally, the 1 -, 3 - and 5-year OS were predicted by establishing the nomogram. The discriminant and predictive ability of the nomogram was evaluated by consistency index (C-index), calibration plot, area under the curve (AUC), and receiver operating characteristic (ROC) curve. Decision curve analysis (DCA) was used to evaluate the clinical value of the nomogram. Results:A total of 3478 patients diagnosed with EPEC were extracted from the SEER database, and the data were randomly divided into the training group (n=2436) and the validation group (n=1402). T stage, N stage, M stage, surgery, chemotherapy, radiotherapy, age, grade, and tumor size were independent risk factors for 1 -, 3 - and 5-year OS of EPEC (P< 0.05), and these factors were used to construct the nomogram prediction mode. The C-index of the validation and training cohorts was 0.718 and 0.739, respectively, which were higher than those of the TNM stage system. The AUC values of the nomogram used to predict 1-, 2-, and 3-year OS were 0.751, 0.744, and 0.786 in the validation cohorts (0.761, 0.777, 0.787 in the training cohorts), respectively. The calibration curve of 1-, 2-, and 3-year OS showed that the prediction of the nomogram was in good agreement with the actual observation. The nomogram exhibited higher clinical utility after evaluation with the 1-, 2-, and 3-year DCA compared with the AJCC stage system. Conclusions:This study shows that the nomogram prediction model for EPEC based on the SEER database has high accuracy and its prediction performance is significantly better than the TNM staging system, which can accurately and individually predict the OS of patients and help clinicians to formulate more accurate and personalized treatment plans.
10.3389/fonc.2022.1008326
A Dynamic Bayesian Model for Breast Cancer Survival Prediction.
IEEE journal of biomedical and health informatics
OBJECTIVE:Predicting breast cancer survival and targeting patients at high-risk of mortality is of crucial importance. METHODS:We built a Bayesian Dynamic Cox (BDCox) model for predicting 5-year overall survival in breast cancer patients using data of the SEER Cancer Registry with 12,840 women. Four feature selection methods were used to identify predictors and enhance parsimony: fast backward variable selection, elastic net, Bayesian Model Average (BMA), and clinical expertise. All resulting models and a baseline full model containing all features were internally validated via bootstrapping and externally validated in the Shanghai Breast Cancer Survival Study. RESULTS:BMA outperformed other feature selection methods in both internal and external validations. The BDCox model with 12 predictors had the best performance. Several predictors showed time-varying associations with survival that are in agreement with previous studies. CONCLUSION:The model developed using BDCox outperformed other prognostic models considered in our study. The internal validation results indicate that the BDCox model is capable of achieving high prediction accuracy (C-statistic: 0.802), and the external validation results showed excellent generalizability of the BDCox model (C-statistic: 0.739). SIGNIFICANCE:We built a dynamic Bayesian model from the large population-based registry SEER for predicting 5-year breast cancer overall survival. The prediction performance of the BDCox model is significantly better than other survival models.
10.1109/JBHI.2022.3202937
Machine learning-based diffusion model for prediction of coronavirus-19 outbreak.
Neural computing & applications
The coronavirus pandemic has been globally impacting the health and prosperity of people. A persistent increase in the number of positive cases has boost the stress among governments across the globe. There is a need of approach which gives more accurate predictions of outbreak. This paper presents a novel approach called diffusion prediction model for prediction of number of coronavirus cases in four countries: India, France, China and Nepal. Diffusion prediction model works on the diffusion process of the human contact. Model considers two forms of spread: when the spread takes time after infecting one person and when the spread is immediate after infecting one person. It makes the proposed model different over other state-of-the art models. It is giving more accurate results than other state-of-the art models. The proposed diffusion prediction model forecasts the number of new cases expected to occur in next 4 weeks. The model has predicted the number of confirmed cases, recovered cases, deaths and active cases. The model can facilitate government to be well prepared for any abrupt rise in this pandemic. The performance is evaluated in terms of accuracy and error rate and compared with the prediction results of support vector machine, logistic regression model and convolution neural network. The results prove the efficiency of the proposed model.
10.1007/s00521-021-06376-x
Establishing a Risk Prediction Model for Atherosclerosis in Systemic Lupus Erythematosus.
Xing Haiping,Pang Haiyu,Du Tian,Yang Xufei,Zhang Jing,Li Mengtao,Zhang Shuyang
Frontiers in immunology
Patients with systemic lupus erythematosus (SLE) have a significantly higher incidence of atherosclerosis than the general population. Studies on atherosclerosis prediction models specific for SLE patients are very limited. This study aimed to build a risk prediction model for atherosclerosis in SLE. RNA sequencing was performed on 67 SLE patients. Subsequently, differential expression analysis was carried out on 19 pairs of age-matched SLE patients with (AT group) or without (Non-AT group) atherosclerosis using peripheral venous blood. We used logistic least absolute shrinkage and selection operator regression to select variables among differentially expressed (DE) genes and clinical features and utilized backward stepwise logistic regression to build an atherosclerosis risk prediction model with all 67 patients. The performance of the prediction model was evaluated by area under the curve (AUC), calibration curve, and decision curve analyses. The 67 patients had a median age of 42.7 (Q1-Q3: 36.6-51.2) years, and 20 (29.9%) had atherosclerosis. A total of 106 DE genes were identified between the age-matched AT and Non-AT groups. Pathway analyses revealed that the AT group had upregulated atherosclerosis signaling, oxidative phosphorylation, and interleukin (IL)-17-related pathways but downregulated T cell and B cell receptor signaling. Keratin 10, age, and hyperlipidemia were selected as variables for the risk prediction model. The AUC and Hosmer-Lemeshow test -value of the model were 0.922 and 0.666, respectively, suggesting a relatively high discrimination and calibration performance. The prediction model had a higher net benefit in the decision curve analysis than that when predicting with age or hyperlipidemia only. We built an atherosclerotic risk prediction model with one gene and two clinical factors. This model may greatly assist clinicians to identify SLE patients with atherosclerosis, especially asymptomatic atherosclerosis.
10.3389/fimmu.2021.622216
A Clinical Prediction Model for Unsuccessful Pulmonary Tuberculosis Treatment Outcomes.
Clinical infectious diseases : an official publication of the Infectious Diseases Society of America
BACKGROUND:Despite widespread availability of curative therapy, tuberculosis (TB) treatment outcomes remain suboptimal. Clinical prediction models can inform treatment strategies to improve outcomes. Using baseline clinical data, we developed a prediction model for unsuccessful TB treatment outcome and evaluated the incremental value of human immunodeficiency virus (HIV)-related severity and isoniazid acetylator status. METHODS:Data originated from the Regional Prospective Observational Research for Tuberculosis Brazil cohort, which enrolled newly diagnosed TB patients in Brazil from 2015 through 2019. This analysis included participants with culture-confirmed, drug-susceptible pulmonary TB who started first-line anti-TB therapy and had ≥12 months of follow-up. The end point was unsuccessful TB treatment: composite of death, treatment failure, regimen switch, incomplete treatment, or not evaluated. Missing predictors were imputed. Predictors were chosen via bootstrapped backward selection. Discrimination and calibration were evaluated with c-statistics and calibration plots, respectively. Bootstrap internal validation estimated overfitting, and a shrinkage factor was applied to improve out-of-sample prediction. Incremental value was evaluated with likelihood ratio-based measures. RESULTS:Of 944 participants, 191 (20%) had unsuccessful treatment outcomes. The final model included 7 baseline predictors: hemoglobin, HIV infection, drug use, diabetes, age, education, and tobacco use. The model demonstrated good discrimination (c-statistic = 0.77; 95% confidence interval, .73-.80) and was well calibrated (optimism-corrected intercept and slope, -0.12 and 0.89, respectively). HIV-related factors and isoniazid acetylation status did not improve prediction of the final model. CONCLUSIONS:Using information readily available at treatment initiation, the prediction model performed well in this population. The findings may guide future work to allocate resources or inform targeted interventions for high-risk patients.
10.1093/cid/ciab598
Risk prediction model in rheumatoid arthritis-associated interstitial lung disease.
Respirology (Carlton, Vic.)
BACKGROUND AND OBJECTIVE:RA-ILD has a variable clinical course, and its prognosis is difficult to predict. Moreover, risk prediction models for prognosis remain undefined. METHODS:The prediction model was developed using retrospective data from 153 patients with RA-ILD and validated in an independent RA-ILD cohort (n = 149). Candidate variables for the prediction models were screened using a multivariate Cox proportional hazard model. C-statistics were calculated to assess and compare the predictive ability of each model. RESULTS:In the derivation cohort, the median follow-up period was 54 months, and 38.6% of the subjects exhibited a UIP pattern on HRCT imaging. In multivariate Cox analysis, old age (≥60 years, HR: 2.063), high fibrosis score (≥20% of the total lung extent, HR: 4.585), a UIP pattern (HR: 1.899) and emphysema (HR: 2.596) on HRCT were significantly poor prognostic factors and included in the final model. The prediction model demonstrated good performance in the prediction of 5-year mortality (C-index: 0.780, P < 0.001); furthermore, patients at risk were divided into three groups with 1-year mortality rates of 0%, 5.1% and 24.1%, respectively. Predicted and observed mortalities at 1, 2 and 3 years were similar in the derivation cohort, and the prediction model was also effective in predicting prognosis of the validation cohort (C-index: 0.638, P < 0.001). CONCLUSION:Our results suggest that a risk prediction model based on HRCT variables could be useful for patients with RA-ILD.
10.1111/resp.13848
Establishing a prediction model for recurrence of condyloma acuminatum.
European journal of medical research
We collected the clinical data of 156 patients diagnosed with condyloma acuminatum (CA), including age, gender, marriage, education level, stay up late, smoking, drinking, number of sexual partners, HPV infection status of sexual partners, genitourinary and anal diseases, condom use, other diseases of HPV infection, location and number of warts, HPV typing, etc. Analyze the risk factors affecting the recurrence of CA, explore the influencing factors and independent influencing factors of CA recurrence, establish the prediction model of CA recurrence, and evaluate its prediction value. Univariate analysis showed that stay up late, HPV infection status of sexual partners, urogenital diseases, condom use, other diseases of HPV infection and the number of CA were the influencing factors of CA recurrence. Multivariate analysis showed that condom use (OR = 0.166), HPV infection status of sexual partners (OR = 4.848), number of warts (OR = 1.212) and urogenital diseases (OR = 3.179) were independent factors affecting the recurrence of CA (P < 0.05). Therefore, the prediction model of CA recurrence can be established, and the area under the curve AUC of the prediction model was calculated to be 0.867 (95% CI 0.812-0.923). The model established in this study has certain prediction value for the recurrence of CA and can be used to preliminarily predict the recurrence of CA.
10.1186/s40001-022-00816-7
Preprocedural Prediction Model for Contrast-Induced Nephropathy Patients.
Yin Wen-Jun,Yi Yi-Hu,Guan Xiao-Feng,Zhou Ling-Yun,Wang Jiang-Lin,Li Dai-Yang,Zuo Xiao-Cong
Journal of the American Heart Association
BACKGROUND:Several models have been developed for prediction of contrast-induced nephropathy (CIN); however, they only contain patients receiving intra-arterial contrast media for coronary angiographic procedures, which represent a small proportion of all contrast procedures. In addition, most of them evaluate radiological interventional procedure-related variables. So it is necessary for us to develop a model for prediction of CIN before radiological procedures among patients administered contrast media. METHODS AND RESULTS:A total of 8800 patients undergoing contrast administration were randomly assigned in a 4:1 ratio to development and validation data sets. CIN was defined as an increase of 25% and/or 0.5 mg/dL in serum creatinine within 72 hours above the baseline value. Preprocedural clinical variables were used to develop the prediction model from the training data set by the machine learning method of random forest, and 5-fold cross-validation was used to evaluate the prediction accuracies of the model. Finally we tested this model in the validation data set. The incidence of CIN was 13.38%. We built a prediction model with 13 preprocedural variables selected from 83 variables. The model obtained an area under the receiver-operating characteristic (ROC) curve (AUC) of 0.907 and gave prediction accuracy of 80.8%, sensitivity of 82.7%, specificity of 78.8%, and Matthews correlation coefficient of 61.5%. For the first time, 3 new factors are included in the model: the decreased sodium concentration, the INR value, and the preprocedural glucose level. CONCLUSIONS:The newly established model shows excellent predictive ability of CIN development and thereby provides preventative measures for CIN.
10.1161/JAHA.116.004498
A Comorbidity Knowledge-Aware Model for Disease Prognostic Prediction.
IEEE transactions on cybernetics
Prognostic prediction is the task of estimating a patient's risk of disease development based on various predictors. Such prediction is important for healthcare practitioners and patients because it reduces preventable harm and costs. As such, a prognostic prediction model is preferred if: 1) it exhibits encouraging performance and 2) it can generate intelligible rules, which enable experts to understand the logic of the model's decision process. However, current studies usually concentrated on only one of the two features. Toward filling this gap, in the present study, we develop a novel knowledge-aware Bayesian model taking into consideration accuracy and transparency simultaneously. Real-world case studies based on four years' territory-wide electronic health records are conducted to test the model. The results show that the proposed model surpasses state-of-the-art prognostic prediction models in accuracy and c-statistic. In addition, the proposed model can generate explainable rules.
10.1109/TCYB.2021.3070227
An early prediction model for chronic kidney disease.
Zhao Jing,Zhang Yuan,Qiu Jiali,Zhang Xiaodan,Wei Fengjiang,Feng Jiayi,Chen Chen,Zhang Kai,Feng Shuzhi,Li Wei-Dong
Scientific reports
Based on the high incidence of chronic kidney disease (CKD) in recent years, a better early prediction model for identifying high-risk individuals before end-stage renal failure (ESRD) occurs is needed. We conducted a nested case-control study in 348 subjects (116 cases and 232 controls) from the "Tianjin Medical University Chronic Diseases Cohort". All subjects did not have CKD at baseline, and they were followed up for 5 years until August 2018. Using multivariate Cox regression analysis, we found five nongenetic risk factors associated with CKD risks. Logistic regression was performed to select single nucleotide polymorphisms (SNPs) from which we obtained from GWAS analysis of the UK Biobank and other databases. We used a logistic regression model and natural logarithm OR value weighting to establish CKD genetic/nongenetic risk prediction models. In addition, the final comprehensive prediction model is the arithmetic sum of the two optimal models. The AUC of the prediction model reached 0.894, while the sensitivity was 0.827, and the specificity was 0.801. We found that age, diabetes, and normal high values of urea nitrogen, TGF-β, and ADMA were independent risk factors for CKD. A comprehensive prediction model was also established, which may help identify individuals who are most likely to develop CKD early.
10.1038/s41598-022-06665-y
MLP-Based Regression Prediction Model For Compound Bioactivity.
Frontiers in bioengineering and biotechnology
The development of breast cancer is closely linked to the estrogen receptor ERα, which is also considered to be an important target for the treatment of breast cancer. Therefore, compounds that can antagonize ERα activity may be drug candidates for the treatment of breast cancer. In drug development, to save manpower and resources, potential active compounds are often screened by establishing compound activity prediction model. For the 1974 compounds collected, the top 20 molecular descriptors that significantly affected the biological activity were screened using LASSO regression models combined with 10-fold cross-validation method. Further, a regression prediction model based on the MLP fully connected neural network was constructed to predict the bioactivity values of 50 new compounds. To measure the validity of the model, the model loss term was specified as the mean squared error (MSE). The results showed that the MLP-based regression prediction model had a loss value of 0.0146 on the validation set. This model is therefore well trained and the prediction strategy used is valid. The methods developed by this paper may provide a reference for the development of anti-breast cancer drugs.
10.3389/fbioe.2022.946329
Machine Learning Prediction Model for Acute Renal Failure After Acute Aortic Syndrome Surgery.
Frontiers in medicine
BACKGROUND:Acute renal failure (ARF) is the most common major complication following cardiac surgery for acute aortic syndrome (AAS) and worsens the postoperative prognosis. Our aim was to establish a machine learning prediction model for ARF occurrence in AAS patients. METHODS:We included AAS patient data from nine medical centers ( = 1,637) and analyzed the incidence of ARF and the risk factors for postoperative ARF. We used data from six medical centers to compare the performance of four machine learning models and performed internal validation to identify AAS patients who developed postoperative ARF. The area under the curve (AUC) of the receiver operating characteristic (ROC) curve was used to compare the performance of the predictive models. We compared the performance of the optimal machine learning prediction model with that of traditional prediction models. Data from three medical centers were used for external validation. RESULTS:The eXtreme Gradient Boosting (XGBoost) algorithm performed best in the internal validation process (AUC = 0.82), which was better than both the logistic regression (LR) prediction model (AUC = 0.77, < 0.001) and the traditional scoring systems. Upon external validation, the XGBoost prediction model (AUC =0.81) also performed better than both the LR prediction model (AUC = 0.75, = 0.03) and the traditional scoring systems. We created an online application based on the XGBoost prediction model. CONCLUSIONS:We have developed a machine learning model that has better predictive performance than traditional LR prediction models as well as other existing risk scoring systems for postoperative ARF. This model can be utilized to provide early warnings when high-risk patients are found, enabling clinicians to take prompt measures.
10.3389/fmed.2021.728521
A Machine Learning-Based Prediction Model for Acute Kidney Injury in Patients With Congestive Heart Failure.
Peng Xi,Li Le,Wang Xinyu,Zhang Huiping
Frontiers in cardiovascular medicine
Background:Machine learning (ML) has been used to build high performance prediction model. Patients with congestive heart failure (CHF) are vulnerable to acute kidney injury (AKI) which makes treatment difficult. We aimed to establish an ML-based prediction model for the early identification of AKI in patients with CHF. Methods:Patients data were extracted from the Medical Information Mart for Intensive Care III (MIMIC-III) database, and patients with CHF were selected. Comparisons between several common ML classifiers were conducted to select the best prediction model. Recursive feature elimination (RFE) was used to select important prediction features. The model was improved using hyperparameters optimization (HPO). The final model was validated using an external validation set from the eICU Collaborative Research Database. The area under the receiver operating characteristic curve (AUROC), accuracy, calibration curve and decision curve analysis were used to evaluate prediction performance. Additionally, the final model was used to predict renal replacement therapy (RRT) requirement and to assess the short-term prognosis of patients with CHF. Finally, a software program was developed based on the selected features, which could intuitively report the probability of AKI. Results:A total of 8,580 patients with CHF were included, among whom 2,364 were diagnosed with AKI. The LightGBM model showed the best prediction performance (AUROC = 0.803) among the 13 ML-based models. After RFE and HPO, the final model was established with 18 features including serum creatinine (SCr), blood urea nitrogen (BUN) and urine output (UO). The prediction performance of LightGBM was better than that of measuring SCr, UO or SCr combined with UO (AUROCs: 0.809, 0.703, 0.560 and 0.714, respectively). Additionally, the final model could accurately predict RRT requirement in patients with (AUROC = 0.954). Moreover, the participants were divided into high- and low-risk groups for AKI, and the 90-day mortality in the high-risk group was significantly higher than that in the low-risk group (log-rank < 0.001). Finally, external validation using the eICU database comprising 9,749 patients with CHF revealed satisfactory prediction outcomes (AUROC = 0.816). Conclusion:A prediction model for AKI in patients with CHF was established based on LightGBM, and the prediction performance of this model was better than that of other models. This model may help in predicting RRT requirement and in identifying the population with poor prognosis among patients with CHF.
10.3389/fcvm.2022.842873
Development and validation of a risk prediction model for anxiety or depression among patients with chronic obstructive pulmonary disease between 2018 and 2020.
Annals of medicine
Anxiety and depression are important risk factors for chronic obstructive pulmonary disease (COPD). The aim of this study was to develop a prediction model to predict anxiety or depression in COPD patients. The retrospective study was conducted in COPD patients receiving stable treatment between 2018 and 2020 to develop prediction model. The variables, were readily available in clinical practice, were analysed. After data preprocessing, model training and performance evaluation were performed. Validity of the prediction model was verified in 3 comparative model training. Between 2018 and 2020, 375 eligible patients were analysed. Thirteen variables were included into the final model: gender, age, marital status, education level, long-term residence, per capita annual household income, payment method of medical expenses, direct economic costs of treating COPD in the past year, smoking, COPD progression, number of acute exacerbation of COPD in the last year, regular treatment with inhalants and family oxygen therapy. Risk score threshold in each sample in the training set was 1.414. The area under the curve value was respectively 0.763 and 0.702 in the training set and test set, which were higher than three comparative models. The simple prediction model to predict anxiety or depression in patients with COPD has been developed. Based on 13 available data in clinical indicators, the model may serve as an instrument for clinical decision-making for COPD patients who may have anxiety or depression.Key messagesThirteen variables were included into the prediction model.The AUC value was, respectively, 0.763 and 0.702 in the training set and test set, which were higher than three comparative models.The simple prediction model to predict anxiety or depression in patients with COPD has been developed.
10.1080/07853890.2022.2105394
A Novel Prediction Model for Brain Glioma Image Segmentation Based on the Theory of Bose-Einstein Condensate.
Zhang Tian Chi,Zhang Jing,Chen Shou Cun,Saada Bacem
Frontiers in medicine
Background:The input image of a blurry glioma image segmentation is, usually, very unclear. It is difficult to obtain the accurate contour line of image segmentation. The main challenge facing the researchers is to correctly determine the area where the points on the contour line belong to the glioma image. This article highlights the mechanism of formation of glioma and provides an image segmentation prediction model to assist in the accurate division of glioma contour points. The proposed prediction model of segmentation associated with the process of the formation of glioma is innovative and challenging. Bose-Einstein Condensate (BEC) is a microscopic quantum phenomenon in which atoms condense to the ground state of energy as the temperature approaches absolute zero. In this article, we propose a BEC kernel function and a novel prediction model based on the BEC kernel to detect the relationship between the process of the BEC and the formation of a brain glioma. Furthermore, the theoretical derivation and proof of the prediction model are given from micro to macro through quantum mechanics, wave, oscillation of glioma, and statistical distribution of laws. The prediction model is a distinct segmentation model that is guided by BEC theory for blurry glioma image segmentation. Results:Our approach is based on five tests. The first three tests aimed at confirming the measuring range of T and μ in the BEC kernel. The results are extended from -10 to 10, approximating the standard range to T ≤ 0, and μ from 0 to 6.7. Tests 4 and 5 are comparison tests. The comparison in Test 4 was based on various established cluster methods. The results show that our prediction model in image evaluation parameters of P, R, and F is the best amongst all the existent ten forms except for only one reference with the mean value of F that is between 0.88 and 0.93, while our approach returns between 0.85 and 0.99. Test 5 aimed to further compare our results, especially with CNN (Convolutional Neural Networks) methods, by challenging Brain Tumor Segmentation (BraTS) and clinic patient datasets. Our results were also better than all reference tests. In addition, the proposed prediction model with the BEC kernel is feasible and has a comparative validity in glioma image segmentation. Conclusions:Theoretical derivation and experimental verification show that the prediction model based on the BEC kernel can solve the problem of accurate segmentation of blurry glioma images. It demonstrates that the BEC kernel is a more feasible, valid, and accurate approach than a lot of the recent year segmentation methods. It is also an advanced and innovative model of prediction deducing from micro BEC theory to macro glioma image segmentation.
10.3389/fmed.2022.794125
Prediction Model Risk-of-Bias Assessment Tool for coronary artery lesions in Kawasaki disease.
Frontiers in cardiovascular medicine
Objective:To review and critically appraise articles on prediction models for coronary artery lesions (CALs) in Kawasaki disease included in PubMed, Embase, and Web of Science databases from January 1, 1980, to December 23, 2021. Materials and methods:Study screening, data extraction, and quality assessment were performed by two independent reviewers, with a statistics expert resolving discrepancies. Articles that developed or validated a prediction model for CALs in Kawasaki disease were included. The Critical Appraisal and Data Extraction for Systematic Reviews of Prediction Modeling Studies checklist was used to extract data from different articles, and Prediction Model Risk-of-Bias Assessment Tool (PROBAST) was used to assess the bias risk in different prediction models. We screened 19 studies from a pool of 881 articles. Results:The studies included 73-5,151 patients. In most studies, univariable logistic regression was used to develop prediction models. In two studies, external data were used to validate the developing model. The most commonly included predictors were C-reactive protein (CRP) level, male sex, and fever duration. All studies had a high bias risk, mostly because of small sample size, improper handling of missing data, and inappropriate descriptions of model performance and the evaluation model. Conclusion:The prediction models were suitable for the subjects included in the studies, but were poorly effective in other populations. The phenomenon may partly be due to the bias risk in prediction models. Future models should address these problems and PROBAST should be used to guide study design.
10.3389/fcvm.2022.1014067
Establishment and evaluation of a prediction model for acute gastrointestinal injury in patients with prolonged disorder of consciousness.
BMC gastroenterology
OBJECTIVE:To establish a prediction model for acute gastrointestinal injury (AGI) in patients with prolonged disorder of consciousness (pDOC) and to evaluate and apply the prediction model. METHODS: The clinical data of 165 patients with pDOC admitted to the hyperbaric oxygen department from January 2021 to December 2021 were retrospectively reviewed, and the patients were divided into an AGI group (n = 91) and an N-AGI group (n = 74) according to whether AGI occurred. A prediction model was built by fitting multiple independent influencing factors through logistic regression. The receiver operating characteristic (ROC) curve was used to evaluate the predictive value of the model, the Hosmer-Lemeshow (H-L) test was used to evaluate the goodness-of-fit of the model, and the ROC curve and calibration curve were drawn to evaluate the predictive performance. A nomogram was plotted to visualize the prediction model. RESULTS:According to the multivariate logistic regression analysis results, the prediction model was finally constructed with the CRS-R score, DAO, PCT, ALB, and I-FABP, and a nomogram was generated. The area under the ROC curve (AUC) of the prediction model was 0.931, the sensitivity was 83.5%, and the specificity was 93.2%. The data were divided into 5 groups for the H-L test (χ = 2.54, P = 0.468 > 0.05) and into 10 groups for the H-L test (χ = 9.98, P = 0.267 > 0.05). A calibration curve was drawn based on the test results, indicating that the prediction model has a good goodness-of-fit and good prediction stability. CONCLUSION:The prediction model for AGI in pDOC patients constructed in this study can be used in clinical practice and is helpful to predict the occurrence of AGI in pDOC patients.
10.1186/s12876-022-02536-y
Prediction of overall survival in resectable intrahepatic cholangiocarcinoma: IS -applied prediction model.
Tian Mengxin,Liu Weiren,Tao Chenyang,Tang Zheng,Zhou Yufu,Song Shushu,Jin Lei,Wang Han,Jiang Xifei,Zhou Peiyun,Fang Yuan,Qu Weifeng,Ding Zhenbin,Peng Yuanfei,Fu Xiutao,Qiu Shuangjian,Zhou Jian,Fan Jia,Shi Yinghong
Cancer science
Intrahepatic cholangiocarcinoma (ICC) remains a highly heterogeneous disease with poor prognosis. Tumor-infiltrating lymphocytes were predictive in various cancers, but their prognostic value in ICC is less clear. A total of 168 ICC patients who had received liver resection were enrolled and assigned to the derivation cohort. Sixteen immune markers in tumor and peritumor regions were examined by immunohistochemistry. A least absolute shrinkage and selection operator model was used to identify prognostic markers and to establish an immune signature for ICC (IS ). An IS -applied prediction model was built and validated in another independent dataset. Five immune features, including CD3 , CD57 , CD45RA , CD66b and PD-L1 , were identified and integrated into an individualized IS for each patient. Seven prognostic predictors, including total bilirubin, tumor numbers, CEA, CA19-9, GGT, HBsAg and IS , were integrated into the final model. The C-index of the IS -applied prediction model was 0.719 (95% CI, 0.660-0.777) in the derivation cohort and 0.667 (95% CI, 0.581-0.732) in the validation cohort. Compared with the conventional staging systems, the new model presented better homogeneity and a lower Akaike information criteria value in ICC. The IS -applied prediction model may provide a better prediction performance for the overall survival of patients with resectable ICC in clinical practice.
10.1111/cas.14315